A data integration strategy is a detailed plan on how an organization combines data from different sources into a single location. The goal is to improve decision-making, operational efficiency, and customer relations. This also drives innovation that places your company in league with the top players in your industry.
There are various key systems managing different data domains in a company. They have a system for marketing, human resources, content management, and more. A data integration strategy aims to form a single source of truth from these systems. This will open possibilities for analysis and business intelligence. In addition, this will improve data consistency across these different systems.
This article will discuss the following:
- The benefits of having a data integration strategy,
- Common challenges,
- Key components needed to complete the strategy,
- Data integration methods to choose from,
- Key steps to achieve an ideal strategy.
We will also consider a sample of what a data integration strategy may look like.
Let’s begin.
Benefits of Having a Data Integration Strategy
Let's dive deeper into what your organization can get when implementing a data integration strategy.
1. Improved Decision-Making
When all key information is in one place, decision-makers can see the full picture. The result is better, data-driven decisions.
Example: A retail company integrates sales, inventory, and customer data from various systems. This allows managers to identify top-selling products, understand customer preferences, and optimize inventory levels. Stock management improves and sales increase.
2. Increased Efficiency and Improved Productivity
This means that processes and workflows improved and became simpler. Without automated data integration, consolidation is manual and takes longer to do. Combining all relevant data with a system reduces manual work and allows employees to focus on other important tasks.
Example: A retail company integrates sales, inventory, and customer data from various systems. This allows managers to identify top-selling products, understand customer preferences, and optimize inventory levels. Stock management improves and sales increase.
3. Enhanced Data Quality
This means data is accurate, consistent, and up-to-date across the organization. There are processes and systems in place to ensure this happens. High-quality data leads to reliable insights and avoids costly mistakes.
Example: A healthcare provider integrates patient records from different departments into a single system. By doing so, they reduced redundant processes and duplicate entries. So, patient information became current and more accurate. This is crucial for patient care and regulatory compliance.
4. Better Customer Insights
This means gaining a detailed view of customer behavior and preferences. Understanding customers leads to better-targeted marketing, improved customer service, and happy customers.
Example: An e-commerce company integrates data from its website, CRM, and social media platforms. This integration provides a 360-degree view of customer interactions. It also paved the way for personalized marketing campaigns and tailored customer support.
5. Cost Savings
Removing manual and redundant processes through data integration improves efficiency and productivity. The result is lower operational costs.
Example: A manufacturing company has various production systems. So, they planned to consolidate their data into a single storage solution. This feeds a system that uses Artificial Intelligence. The addition of AI improved productivity and eventually cost savings.
6. Regulatory Compliance
An effective data integration strategy ensures auditable data for regulatory compliance. Compliance avoids legal issues and fines while maintaining trust with customers and stakeholders.
Example: A financial firm integrates data from various departments. This enables tracking and reporting all transactions based on regulatory requirements. The integration helps the institution quickly respond to audits and comply with regulations like GDPR and SOX.
7. Scalability
Scalable data integration solutions can handle increasing data volumes as the business expands. So, it is crucial to design the integration solution to be scalable to avoid performance issues.
Example: A fast-growing tech startup implements Skyvia, a scalable cloud-based data integration platform. The platform scales to accommodate the increased load without performance issues. So, the business can expand and innovate even if data keeps growing.
Common Challenges in Implementing a Data Integration Strategy
An ideal data integration strategy cannot happen overnight. Some stumbling blocks may have been in place before it even started. Here are some of the most challenging ones:
Data Silos
These are data kept by users in the organization that are isolated and impossible to integrate. Users may not want to abandon them. This leads to incomplete insights. So, decision-makers are in the dark to see the big picture.
Example: A multinational company has various sales regions across the world. But these regions have their own databases that do not communicate with each other. So, global sales trends are a pain to analyze which hampers strategic decision-making.
Data Quality Issues
Poor data quality leads to questionable insights that eventually lead to wrong decisions. It is a best practice to clean data by removing duplicates, incomplete entries, and the like.
Example: A big hospital wants to integrate patient data from various departments. But each department holds different data formatting. For example, date and number formats are different. They are also duplicated as the patient transacts with each department. Cleaning the data during data integration is a tremendous challenge.
Integration Complexity
Some factors increase data integration complexity. Among them are proprietary data formats, close architecture, and large data volumes. Complex integration processes can be prone to errors, consume a lot of time, and be expensive.
Example: An insurance firm decides to integrate new technology solutions with legacy systems. But the proprietary data format requires many customizations. This delays the project and increases costs.
Data Security and Privacy
New threats can come anytime, and regulations can change. That is why securing the data and complying with regulations becomes a challenge. Data breaches and non-compliance can damage company's reputation and lead to severe financial burdens.
Example: An investment company stores sensitive customer information across different systems. There is a mobile and web app facing their customers. The data from the apps is integrated into a CRM and accounting solution. To avoid data breaches and ensure compliance with GDPR, the company ensures the latest encryption methods, security protocols, and access controls are in place and maintained. But their small technical team finds it hard to keep up.
Scalability Issues
An inadequate data integration solution may run slower as data increases in size. It will lead to loss of productivity and inefficiency. Sometimes, it may also lead to data loss.
Example: An e-commerce website experiences rapid growth. The online retailer finds their data integration solution can no longer scale. The results are outdated insights and reports, and disgruntled customers.
High Costs
Data integration projects need a financial investment in technologies, tools, and skilled staff. Project issues can further increase costs. High costs can strain budgets and limit the scope of data integration projects.
Example: A financial firm integrates data from various departments. This enables tracking and reporting all transactions based on regulatory requirements. The integration helps the institution quickly respond to audits and comply with regulations like GDPR and SOX.
Change Management
This is the difficulty of managing organizational changes and getting buy-in from all stakeholders. Some users are resistant to change. So, adopting new systems and processes including data integration becomes a challenge.
Example: An agency providing human resource services desires to implement a data integration strategy. But some employees prefer the old way of doing things and become a showstopper to the project.
Key Components in a Data Integration Strategy
When forming a data integration strategy, key components play a crucial role on how effective the strategy can be. Here they are:
1. Data Sources
These are the origins of your data, like databases, cloud services, applications, and external APIs. Knowing where your data comes from helps you plan how to gather and integrate it.
2. Goals
Goals are what you want to achieve with your integrated data. Clear goals are the compass of your strategy. It ensures stakeholders are on the same page and working for the same purpose. It also sets the boundaries of the entire data integration strategy.
3. Tools and Technologies
These are the software and platforms to collect, transform, and store data. Choosing the right tools ensures your integration process is efficient, scalable, and reliable.
4. Data Quality
These are the processes and tools to make data accurate, consistent, reliable, and up-to-date. Data quality is usually handled after extraction from the data sources. The process should include only complete and non-duplicate data before it reaches the target repository.
5. Security and Compliance
Special tools and processes aim to protect your data from unauthorized access and ensure you follow legal and regulatory requirements. This involves both data-at-rest and data-in-transit.
6. Stakeholders
People involved in the overall data integration strategy can be business leaders, IT staff, data analysts, and end-users. It is always a good idea to involve them at the beginning to make sure the strategy aligns with business goals and satisfies the needs of everyone. It allows for smooth adoption and reduces resistance from users.
Data Integration Methods to Consider in Your Strategy
Part of the data integration strategy is the data integration methods or approaches. The chosen approach should fit with goals, tool selection, and other key components.
Depending on the defined goals by key stakeholders, IT specialists will choose the tools and technology to ensure data quality, security, compliance, and scalability. They also have to decide the best approach to achieve the best outcomes.
The following are the different methods to choose from:
1. ETL (Extract, Transform, Load)
-
This is a batch process to pull data from various sources, then clean, format, and combine datait to a usable form. Finally, it stores the transformed data into a target system.
-
Tools: Skyvia, Talend, and Apache Nifi.
-
Use Case: Suitable for batch processing where data is processed in bulk at scheduled times.
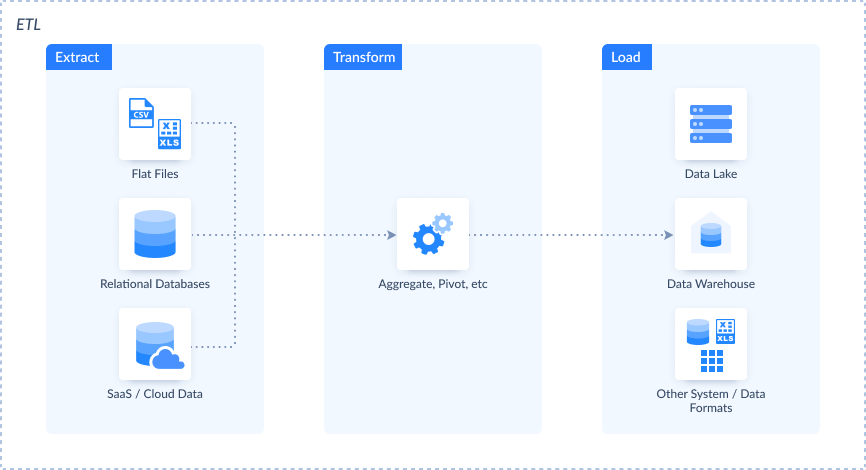
2. ELT (Extract, Load, Transform)
-
Pull data from various sources then directly load raw data into the target system. Perform transformations within the target system afterward.
-
Use Case: Ideal for cloud environments with powerful processing capabilities where transformations are done after loading.
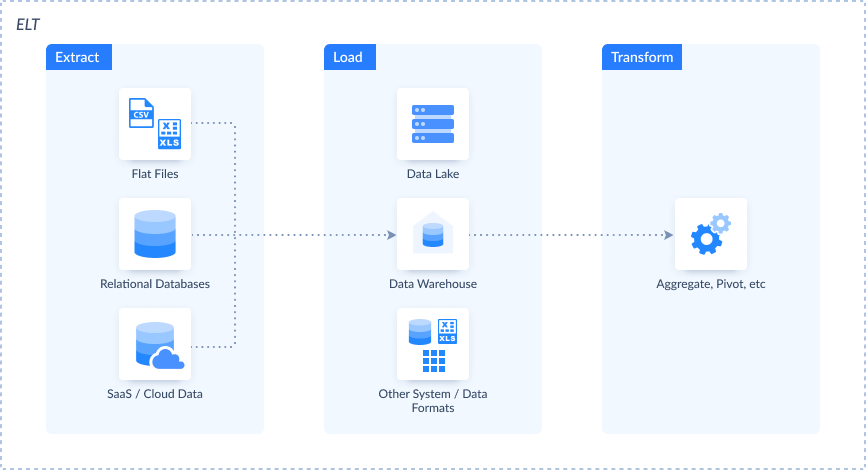
3. Data Streaming
-
Data is processed in real time as it flows from the source to the target.
-
Tools: Apache Kafka and Amazon Kinesis.
-
Use Case: Suitable for applications that require real-time analytics or monitoring.
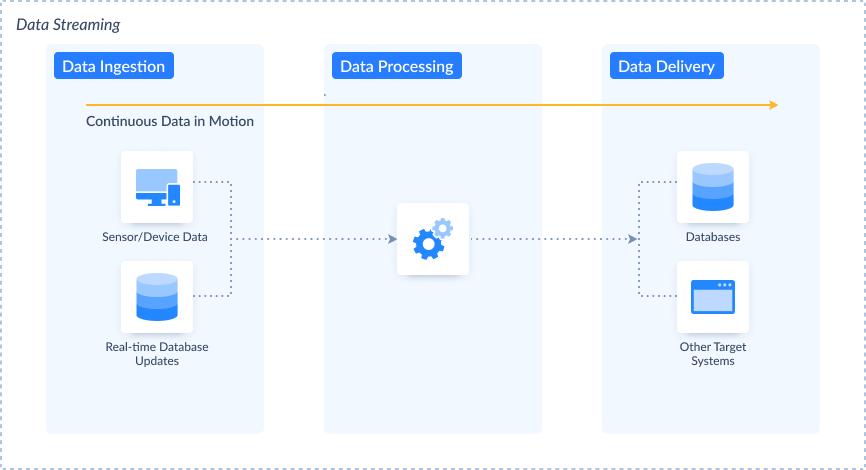
4. Data Virtualization
-
Create a virtual layer that allows querying and manipulation of data from multiple sources without physical consolidation.
-
Tools: Denodo and IBM Cloud Pak for Data.
-
Use Case: Ideal for scenarios where data remains distributed but needs to be accessed and integrated on demand.
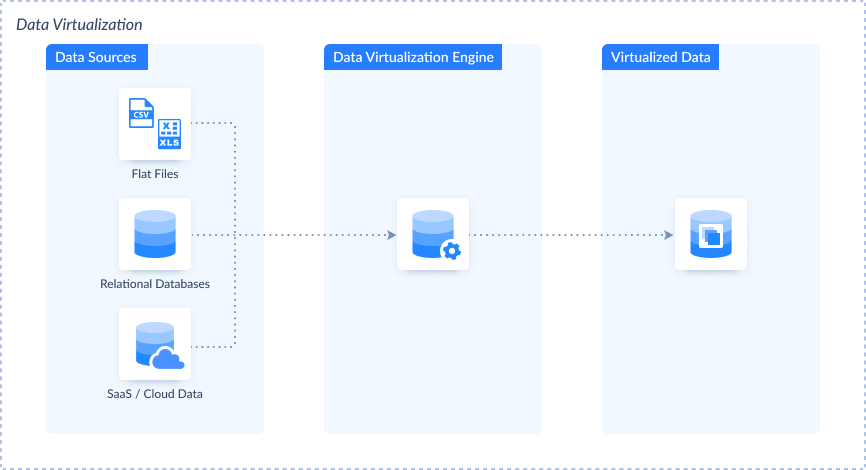
5. Data Replication
-
Periodically copy data from one location to another.
-
Types: Full replication, partial replication.
-
Use Case: Full replication, partial replication.
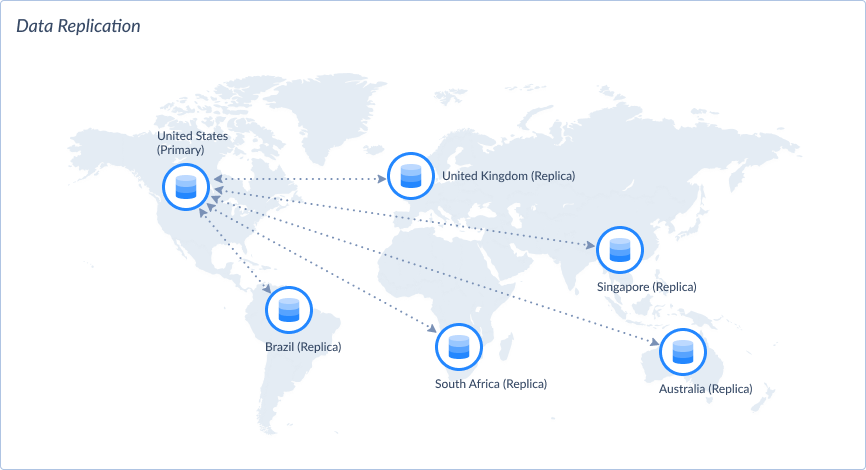
6. API Integration
-
Use Application Programming Interfaces (API) to connect and integrate different systems and applications
-
Use Case: Useful for integrating applications and services in a flexible and scalable manner.
Choosing the Right Approach
-
ETL vs. ELT: Choose ETL for traditional data warehousing with complex transformations before loading. Opt for ELT if using cloud data warehouses with powerful in-database processing capabilities.
-
Real-Time vs. Batch: Use data streaming for real-time needs (e.g., live dashboards, alerts). Use batch processing for large-scale, periodic data updates.
-
Virtualization vs. Physical Integration: Use data virtualization to avoid data redundancy and provide real-time data access. Choose physical integration like data streaming, ETL, or ELT when performance and data consolidation are critical.
-
Hybrid: Depending on requirements, a mix of methods can be used. For example, alerts and live dashboards will use data streaming while others may benefit from ETL, ELT, or data virtualization.
By understanding these methods, you can design a robust data integration architecture that meets your specific needs and supports your overall strategy.
Steps to Implement a Data Integration Strategy
The following are the key steps to implement an ideal data integration strategy:
1. Assessment
This step outlines the process and resources needed, guided by the defined scope and purpose. This also includes the major milestones, activities, schedules, and costs that align with objectives.
-
Key Components Involved:
- Data Sources: Identify where your data is coming from.
- Stakeholders: Engage key stakeholders to understand their needs and expectations.
-
Example: Conducting a data audit, defining that the project aims to integrate customer data from CRM and sales systems, and involving business leaders to set the project's objectives.
2. Planning
This step outlines the process and resources needed, guided by the defined scope and purpose. This will also include the major milestones, activities, schedule, and costs that aligns with objectives.
-
Key Components Involved:
- Goals: Define what you want to achieve.
- Stakeholders: Collaborate with stakeholders to ensure the plan meets their needs.
-
Example: Developing a roadmap with clear steps, timelines, and responsibilities. Ensure the plan is aligned with business leaders' strategic goals.
3. Tool Selection
This is picking the right software and tools for your integration needs. At this point, the data integration method(s) are also chosen to ensure the tools match your defined scope and goals. They should also meet budget constraints.
-
Key Components Involved:
- Tools and Technologies: Select the right tools for data extraction, transformation, and loading.
- Stakeholders: Get input from IT staff and data analysts on tool selection.
-
Example: Choosing ETL tools that can handle the specific types of data and transformations needed for the project.
4. Implementation
This step involves executing the plan starting from the pilot project until the entire goal is achieved. The process follows an iterative or phased approach to minimize issues and address them accordingly.
-
Key Components Involved:
- Tools and Technologies: Use the chosen tools and technologies for each project.
- Data Quality: Ensure data is accurate, consistent, and reliable during the process.
- Security and Compliance: Implement security measures in the data integration processes.
- Stakeholders: IT staff and data analysts work on the data integration pipelines using the chosen tools. Other stakeholders are informed on the status based on agreed iteration meeting schedules. Trainings are also provided after every go-live of a new phase.
-
Example: Extracting data from chosen sources, transforming it as planned, and loading it into your target system, while continuously validating data quality.
5. Monitoring and Maintenance
This is a step to continuously check and improve the implemented integration processes. It makes sure the data pipelines run smoothly. At the same time, fixes are made when issues arise based on user feedback.
-
Key Components Involved:
- Security and Compliance: Protect your data and ensure regulatory requirements are met.
- Stakeholders: Collect feedback from end-users and other stakeholders to make improvements. Meanwhile, the technical team will make the adjustments and implement the changes.
-
Example: Regularly reviewing data quality and system performance, setting up automated monitoring to detect errors, and making adjustments as needed based on feedback.
Data Integration Strategy Example
The following is an excerpt of what a data integration strategy looks like in the real world using a fictitious company. The strategy can be a paper document with signatures or a digital one with electronic approvals.
Data Integration Strategy for A-Z Retail Corporation
1. Overview
-
Scope: Integration of customer data from CRM, sales, and marketing systems into a data warehouse.
-
Purpose: To create a unified customer view to improve decision-making and customer service.
-
Goals: Enhance data quality, streamline data access, and support real-time analytics.
2. Stakeholder Analysis
-
Business Leaders: Define strategic goals.
-
IT Staff: Implement and maintain technical solutions.
-
Data Analysts: Validate data quality and usability.
-
End-Users: Provide insights on data usage.
3. Data Inventory
-
CRM System: Salesforce.
-
Sales System: Custom-made web and mobile app using PostgreSQL as the database.
-
Marketing System: HubSpot.
4. Architecture Design
-
ETL Processes: Data extracted from Salesforce, PostgreSQL database, and HubSpot, transformed, and loaded into a Snowflake data warehouse.
-
Data Flow Diagram:
5. Tool Selection
-
ETL Tool: Skyvia – chosen for ease of use and flexible ETL pipeline development. It also seamlessly connects to Salesforce, PostgreSQL, HubSpot, and Snowflake.
-
Data Warehouse: Snowflake – selected for scalability and performance.
6. Data Governance Plan
-
Quality Checks: Regular audits on a schedule and automated validation rules.
-
Security Measures: Data encryption, role-based access control, and tools to use.
-
Compliance: GDPR and HIPAA guidelines adherence.
7. Implementation Plan
-
Phase 1: Data extraction setup (Q1 2024)
-
Phase 2: Data transformation processes (Q2 2024)
-
Phase 3: Data loading and initial testing (Q3 2024)
-
Phase 4: Full deployment and user training (Q4 2024)
8. Monitoring and Maintenance Plan
-
Automated Monitoring: Name the tools to detect and alert on errors.
-
Regular Reviews: Monthly review meetings to assess data quality and integration performance.
9. Risk Management
-
Data Loss: Define backup schedule and redundancy, and the tools needed.
-
Compliance Issues: Regular compliance checks and updates (Include details depending on regulatory body).
Conclusion
In summary, a company desiring to integrate the data from its various systems needs a viable strategy. This documented plan has the goal of improving operational efficiency, decision-making, and customer relations.
While there are issues in data integration projects, having the right strategy ensures goals are achieved. The components, steps, and methods presented here are your basic guide on what to include in your data integration strategy. Cloud data platforms like Skyvia are also crucial to implement the data integration methods chosen.
We hope this article including the example will help any organization to form their data integration strategy.